
Vianney Taquet
I have developed a gas-grain astrochemical model, called GRAINOBLE since 2010. It was first developed to distinguish the physical and chemical processes occurring on the surface of ices and inside the ice mantle and now includes many gas phase reactions and gas-grain processes. A surface chemical network was built following the results of various laboratory experiments and it includes a state-of-the-art gas phase chemical network based on the KIDA database and supplemented by other reactions. The following paragraphs describe a bit more the main specificities of the code.
A public version of GRAINOBLE will be soon available on GitHub.
Multiphase treatment
​
GRAINOBLE now adopts the "3-phase" method introduced by Hasegawa & Herbst (1993). This method allows us to 1) predict the chemical stratification of interstellar ices during their formation in cold dense cores; 2) follow the thermal processing, including evaporation, of ices during their warm-up around protostars.
​
For our study published in Taquet et al. (2014), GRAINOBLE has been applied to a one-dimensional physical model of protostellar formation in order to predict the formation and the evolution of interstellar ices in dense cores and young protostars. As seen on the Figure, the slow formation of interstellar ices in dark clouds induces a strong chemical heterogeneity in the ice mantles. Water, carbon dioxide, and methane are abundant in the inner parts of the ices, while the outer parts of ices are rather composed of carbon monoxide, formaldehyde, and methanol, formed for dense and cold conditions. Such a modelling allows us to explain infrared observations of ices towards dense clouds and protostars by space observations like Spitzer.
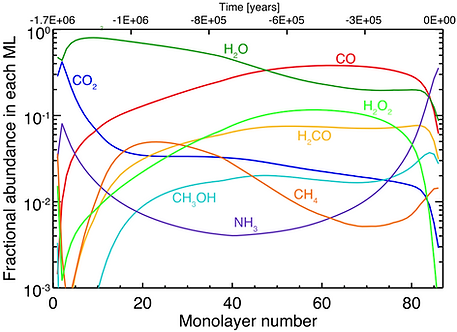
Figure taken from Taquet et al. (2014) presenting the chemical composition inside interstellar ices at the end of the formation of a prestellar core. The fractional abundance of each monolayer is given, the deepest ice layer close to the refractory grain being monolayer 0, while the outermost layers in interaction with the gas are on the right.
Reactivity of surface reactions​
​
A model from quantum chemical methods, the Eckart model, that gives an estimate of the transmission probability of many surface reactions has been introduced in Taquet et al. (2013). From three parameters (the forward and backward activation energies and the frequency of the imaginary mode of the transition state), GRAINOBLE uses the Eckart model to estimate the transmission probability of about 80 reactions included in the chemical network of (deuterated) methanol, water and carbon dioxide.
Impact of surface and chemical parameters on the ice composition​
​
Surface chemical modelling is based on a high number of input parameters. Given the heterogeneity of ices and the complexity of the processes at the surface and in the bulk, the values of most of these parameters are either highly uncertain or show large distributions of values. GRAINOBLE allows us to investigate the impact of these uncertainties on the chemical composition of interstellar ices through the calculations of model grids in which the values of input parameters are varied. An example is shown in the bottom figures. For a study published in Rimola et al. (2014), GRAINOBLE was used to investigate the impact of grain surface parameters (diffusion and binding energies, grain size) and of dust temperature on the abundance of formaldehyde H2CO and methanol CH3OH in ices. The transmission probabilities of the CO+H and H2CO+H reactions have been computed with the Eckart model following three different studies. The three distributions of H2CO abundances induced by the variation of surface parameters are shown in the figure. We have therefore shown that the quantum calculations carried out by Rimola et al. (2014), shown in blue, give the best agreement with observations.
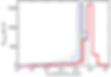
Figure taken from Rimola et al. (2014) presenting the ice abundances of H2CO with respect to water predicted by GRAINOBLE. Standard blue lines show the distributions using the water cluster calculations of Rimola et al. (2014), thin green lines correspond to the gas-phase calculations by Peters et al. (2013), and red thick lines correspond to the ice measurements by Fuchs et al. (2009). Gray boxes show the observed abundances summarized by Oberg et al. (2011) toward low-mass protostars and dark cores.
Application to physical models​
​
GRAINOBLE uses the rate equations formalism, allowing us to follow the chemical evolution of sophisticated chemical networks in a reasonable amount of time. The code can therefore be applied to physical models to follow the chemical evolution both temporally and spatially, in the gas and in ices, of astronomical sources. So far, it has been applied to a simple 1D physical model of core formation and collapse described in Taquet et al. (2014). The bottom figure shows the spatial profile of the gas phase abundances for the most abundant species formed in cold interstellar ices around a typical protostar. It can be noticed that the multilayer treatment adopted in the code induces complex abundance profiles for volatile species such as CO, CO2, or H2CO with double abundance jumps.
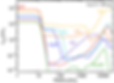
Figure taken from Taquet et al. (2014) presenting the gas-phase abundance profiles of the main ice components obtained for the collapsing core model A-F at the beginning of the Class 0 phase.